Introduction
In 1956 computer scientist John McCarthy coined the phrase ‘artificial intelligence’ (AI) to describe ‘the science and engineering of making intelligent machines’ (McCarthy, 2007). Over time, the term has evolved to cover a variety of technologies, including ones widely used in education, from plagiarism detectors to voice-activated virtual assistants leveraged to enhance campus information distribution and classroom pedagogy (Arizona State University, 2018).
Contemporary AI discussions are about ‘a variety of methods and tools which mimic cognitive functions across three areas: 1) perception/ vision, 2) speech/language, and 3) learning analysis’ (Family Online Safety Institute, 2019). Experts further distinguish between ‘narrow AI’ and ‘artificial general intelligence’. Narrow AI performs well in discrete tasks – think of algorithms that are good at playing chess but wouldn’t help someone drive a car, and vice versa – and is currently having a big impact on society. By contrast, artificial general intelligence can learn across domains and think for itself, possibly in astonishingly more sophisticated ways than humans do. For now, artificial general intelligence is the stuff of science fiction and unresolved debates rage over whether it will ever be created.
Presently, the advancement of AI is associated with machine learning and its subset deep learning. Machine learning involves algorithms using statistics to computationally identify patterns in data sets that are often so large they are referred to as big data (Emerging technology from the arXiv, 2013). Big data is the foundation on which modern AI is built. This vast quantity of data gives scientists and statisticians more opportunities to create AI that can identify increasingly intricate and nuanced patterns. Since these patterns are frequently about how humans think and act, they can be used to make sense of present human behaviour. For example, AI can analyse student facial expressions to infer whether students are bored or engaged while doing an assignment. AI can also be used to more accurately predict future human behaviour. For example, it might be used to estimate which students will be academically successful and which candidates will perform best at a job by scanning for certain keywords in a resume that match or are similar to the resumes of current successful accepted students or employees when they were initially hired. Without big data, these AI applications would not exist. In this article, we use the term AI to refer not only to the definition provided in the previous paragraph, but also to the infrastructure that is used to create the AI, which includes big data, statistical modelling, and machine learning.
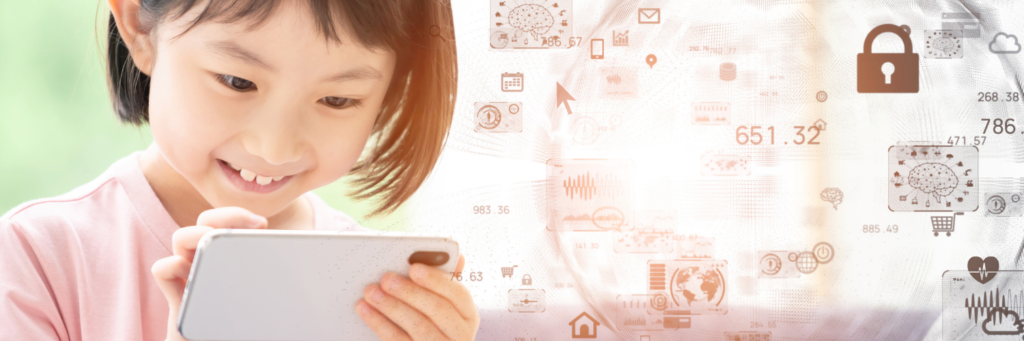
Because this article focuses on privacy and ethics issues in K-12 education, we won’t get into the specific details of technical discussions about AI. Instead, our goal is to identify practical educational uses of tools and systems associated with AI and big data. We will offer clear principles for responsibly using AI and its related big data in educational settings, and make recommendations for how instructors can effectively teach students about the ethical and privacy risks associated with AI and big data in and beyond the educational context.
Although we won’t review any products, from the start we would caution educators and administrators to use care when selecting technologies that are marketed as powered by AI. As discussed earlier, the term AI can encompass a variety of technologies and be interpreted in many ways. Journalistic coverage of AI products and services has been riddled with misinformation. Consequently, companies have enough wiggle room to manipulatively peddle their wares and abuse the term to create exaggerated impressions of how cutting-edge their products are (Marcus, 2019).
How can educators meet the AI imperative?
To put it mildly, high hopes exist for AI. Beyond widely-covered victories of AI over human champions in games requiring immense knowledge, skill, and creativity, such as IBM Watson in Jeopardy!, Google AlphaGo in Go, and AlphaZero AI in chess, AI is being adopted in a range of domains, from medical to military applications to the educational sector. One AI-powered educational testing system is so advanced that it scored over 90% on the multiple-choice questions found on the eighth grade standardised science exam administered in New York State (Clark et al., 2019).
Experts believe AI will significantly impact how teachers instruct, how students engage with learning, and how administrators set priorities and select policies. Consider the following thought experiment about an advanced AI tutor. The scenario has a few distinctive features: it takes place in the future where technological capabilities are deployed that don’t yet exist, it envisions university students learning in virtual classrooms and, crucially, it represents what some in the education technology sector consider a positive step forward.
“The AI tutor will design personalised learning plans that optimise each student’s outcome. Should one student watch their lecture at breakfast time, or in the evening? Where should their first test pop up in a busy schedule? How much preparation will they need to understand a certain concept? While a skeleton crew of humans would be needed initially to design curriculums (the creative bit) and film lectures (CGI is still too expensive), AI tutors could do the rest” (Haw, 2019).
Regardless of debates about whether the scenario described above is desirable or advantageous for students, the most important pedagogical tasks related to AI don’t simply keep humans in the loop, but at the centre of the action. Educators must figure out how to best ensure students are prepared to succeed in a world where AI is deeply embedded in infrastructure and its use influences what jobs are available, what civic life is like, how markets function, and how people communicate and socialise.
Simply put, 21st century education requires preparing students for an AI-intensive future. Educators in primary and secondary schools need to create new lessons and experiment with new educational technologies that can help them meet the challenge of the AI imperative.
The AI imperative is the requirement that educators adequately understand what AI is and the key privacy and ethical issues surrounding its use, effectively teach grade-appropriate versions of that knowledge, and put AI tools to good use in and around the classroom. Failure to meet the AI imperative will leave students unprepared for the increasingly AI-reliant real world. With the stakes so high, the pressure to meet the AI imperative eventually will come from everywhere: from parents, politicians, employers, technology companies, and even the students themselves.
What does it take to meet the AI imperative? Clearly, technical proficiency is essential. For example, educators will need to teach students what algorithms are and gain experience creating programs that involve algorithmic problem-solving and computational thinking. However, this is not enough: without due consideration of privacy and ethics, world-class computer science lessons on machine learning and other forms of AI will fall short. Technology companies themselves acknowledge that this is the case by publishing company-backed ‘AI principles’ that outline vision statements infused with ethical values and commitments (Future of Privacy Forum, n. d.). Research institutions and public sector organisations also recognise the importance of privacy and ethics by proposing principles and guidelines for ethical AI applications (Jobin et al., 2019).
These formal initiatives are also driven by headline-grabbing controversies that have raised the public’s consciousness about the importance of algorithmic accountability. For example, the Australian Online Compliance Intervention program, colloquially referred to as Robodebt, found inconsistencies between Australian Taxation Office income and welfare payments. The program was deployed to generate debt notices and secure ‘up to $4.5 million in Centrelink debt a day’ (Dean, 2019). After, after ‘tens of thousands of welfare recipients’ claimed they were ‘overcharged for alleged debts,’ the federal government decided to change the program. As initially implemented, the program left the burden on wrongly targeted recipients to prove they were treated unfairly (Karp, 2019).
Now, human officers are no longer permitted to exclusively rely on the automated system and are required to conduct ‘further investigations to determine whether a debt exists’ (Marsh, 2019). Mandating human oversight over important decisions is a recurring concern expressed in debates over AI accountability.
Without proper human oversight, education-based AI initiatives can also result in privacy and ethical violations. Respecting student privacy should be a critical component of any effort to meet the AI imperative. Protecting student privacy is not only the right thing to do – it can be legally required. And, because promising programs can be destroyed by poor privacy optics, respecting privacy is instrumentally valuable. If schools or schooling systems communicate poorly with parents about the privacy precautions they are taking and the privacy policies they are following, they may shoulder some of the responsibility if adverse outcomes follow. Opportunities that are genuinely good for students can be misconstrued as dangerous and get shut down if judged irresponsible.
Meeting the AI imperative also requires educators to teach students about the ethical risks of big data and AI systems. Without this knowledge, students won’t be prepared to understand and navigate an increasingly AI-centric world. Quality approaches to teaching ethical risks, including privacy pitfalls, require educators to practice what they preach. If students and parents believe that educators are not modelling good behaviour, their authority could be undermined.
A key lesson that educators should stress is that the most basic way AI will change society is through the choices people make about which AI systems to adopt and reject, and how to wisely use the ones that are selected. By acting as if the AI imperative requires fatalistically acquiescing to a contingently popular technological trend, people neglect their agency and abdicate basic responsibility.
It is simple for educators to rationalise away accountability by acting as if a technologically determined pathway exists, mandating how students must learn. For example, a group of students expressed discomfort about being required to use a classroom engagement detection system that scans students’ faces and uses AI to infer whether they are engaging with material in an attentive manner. When asked to comment on this, the CEO of the company that produced the system bluntly replied that the students’ concerns would not change anything; channelling Darwin, he noted “[e]verybody is doing this. It's really early and shocking, but we cannot go against natural laws of evolution” (Eveleth, 2018).
What a striking response! Starting with the age-old question, “Would you jump off a bridge just because everyone else is doing it?”, we teach young children that something is not necessarily right just because other people are doing it. But adults may fail to heed their own advice when the AI imperative seems to only provide two options: accommodate and adapt all potential applications of AI or perish and give up hope of being relevant.
Technological trends are not natural laws that force individuals and institutions to go in any particular direction. When people, including students, articulate justified ethical reservations that push back against popular positions, seriously considering their concerns can help us forge a better future. Educators should not lose sight of this fact by acting as if they are powerless to contribute to decisions about how to go about meeting the AI imperative.
In the case of the automated engagement detection systems, it is wrong to automatically dismiss student concerns. Without seriously considering the merit of their criticisms, it is impossible to identify students’ values, whether the values deserve the attention of educators and administrators, and whether greater emphasis on these values can motivate the educators using these systems to meet their pedagogical goals through better alternatives.
Students who are dismayed about AI surveillance and analysis in the classroom are often mirroring broader concerns in societal controversies about policing and workplace dynamics. Giving concerned students the impression that advocating for privacy is passé and that authorities should be given unrestricted power over their lives diminishes student agency and threatens their autonomy.
Ingrained privacy and ethics guardrails
We’ve already provided some of the reasons why student privacy should be respected. But let’s think about this issue on a more fundamental level. Clearly, students aren’t entitled to absolute privacy. For safety reasons, teachers and administrators might be justified in searching a locker or a student’s bag for drugs or responding to threats made over social media. If disciplinary problems arise in schools, educators can be obligated to contact parents rather than keep the information confidential. Nevertheless, there are many reasons why students deserve to have some privacy interests protected.
Students are a vulnerable population. Consequently, lack of adequate privacy safeguards can lead to students experiencing embarrassment and shame, even trauma, and suffering lasting reputational damage that can unfairly damage or diminish their future opportunities. Insufficient privacy protections also can lead to exploitation; that’s why many believe that technology companies providing educational services should be prevented from benefitting in any way from student data, even from using it to improve their products. Inadequate privacy measures can also have a chilling effect, inhibiting student self-expression. This harm can impede learning because students who are anxious about privacy issues in schools may have difficulty focusing on scholastic tasks, like lectures and exams. In turn, this can compromise their grades and intellectual maturation. Furthermore, the chilling effect can compromise students’ ability to socialise.
Socialisation is critical to the healthy development of students that schools are supposed to foster. Since schools are responsible for preparing students to become citizens, schools should strive to empower them to think critically about when it is appropriate or inappropriate for authorities, like government officials, employers, and technology companies to engage in privacy diminishing activities like surveillance. If students and parents believe that schools are not adequately protecting their privacy, they might intervene in ways that compromise, if not shut down entirely, promising educational programs.
Since schools should teach students how to grow into responsible, autonomous adults, they should avoid smothering forms of monitoring that lead students to abdicate responsibility for their own behaviour as well as avoid forms of surveillance that will inhibit students from full participation in educational activities. Ideally, schools will provide students with tools for having thoughtful conversations and making thoughtful decisions about privacy in both analogue and digital spaces with their parents, teachers and peers.
For the reasons just provided, it is difficult to neatly distinguish between privacy and ethics. Many privacy protections are rooted in ethical ideals, like respecting autonomy and fostering personal and social development. A useful way to think about the relationship between privacy and ethics is to revisit what happened when data digitisation began in the 1970s. At that time, government officials and policymakers expressed concern, often ethically-minded, about the implications the innovation would have on privacy. Worldwide, committees and commissions were formed to determine the rules for public and private entities that collect, process and share computerised data.
These efforts culminated in the Organisation of Economic Cooperation and Development (OECD) releasing the ‘OECD Guidelines on the Protection of Privacy and Transborder Flows of Person Data’ in 1980, drafted by a group chaired by the then-Chairman of the Australian Law Reform Commission (Gellman, 2019). The guidelines included eight core ideas (paraphrased below) that now lie at the foundation of many privacy laws and proposals around the world. Australia adopted them in 1984, and today these established principles can help provide privacy and ethical guardrails for K-12 institutions to responsibly adopt AI (Greenleaf et al., 2013).
- Information collection should be It should be collected in a fair way, ideally with the knowledge or consent of the person whose data it is.
- Information needs to be accurate, complete, and up-to-date.
- Information should only be collected for a specific purpose that is clearly specified before collection The information should not be reused later in ways that are incompatible with the original specific purpose.
- Information should not be shared or reused in ways incompatible with the original specific purpose without either consent of the person whose data it is or a law providing the authority to do so.
- Information should be protected through reasonable security safeguards that limit the risk of an unauthorised person accessing, using, changing, sharing, or destroying that information.
- There should be transparency about how information is collected, used, shared and protected.
- People have certain rights about their own information, including the right to know what information others have about them, who has it, and the right to request that information be corrected, amended or erased.
- Those holding data must be held accountable for the above principles.
To see how these principles matter in practice, we offer two case studies where privacy and ethical missteps compromised potentially positive advancements in data sharing and educational technology.
Case Study 1 – inBloom
In Australia, inBloom might not be a well-known initiative. However, its rise and fall fundamentally shaped the educational technology landscape in the United States. Even though it is not a cautionary tale about the use of AI, the manner in which the debacle unfolded holds significant lessons for educators everywhere who want to responsibly use and communicate about new educational technologies, particularly in contexts where big data is involved. Indeed, cases like inBloom are especially important to consider now in the early days of using AI in education. Some of the clearest lessons about privacy and ethics can be learned by critically analysing predecessor cases that raise similar issues.
InBloom was a $100 million non-profit educational technology initiative that ran from 2013 to 2014 and aimed to improve American schools by providing a centralised platform for data sharing, learning applications and curricula (Bulger et al., 2017). At the time, many education reformers and student advocates argued that education data should be democratised, and that students should be able to ‘carry’ their data with them from grade-to-grade and post-graduation in a ‘digital backpack’. Despite lofty ambitions, scholastic digital data systems adopted in the 1990s and 2000s were clunky (Denver Post, 2016). Sometimes, data was not even transferable between schools in the same state. Moreover, the systems were often built around minimally useful data points that were used to evaluate students and educators at a single moment in time, instead of continually throughout the year, such as grades and test scores rather than digital portfolios.
The data inBloom could store and the technical architecture of the software were portrayed as game-changers. InBloom was developed to provide secure, semi-instantaneous access to student information, able to facilitate personalised learning, identify learning issues before they manifested in failing grades, provide students and their parents easy access to their own continually updated data, and, overall, help create a more data-driven educational system. Many of these objectives underlie the current push for schools to take advantage of big data and AI.
Soon after inBloom launched, it secured contracts with several states and school districts (Bulger et al., 2017). For many parents, inBloom’s launch was the first time they heard about how data collection and use in the educational sector had changed since the passage of the major U.S. education law, No Child Left Behind (NCLB). NCLB required schools to collect more data to ensure that all students were being served equally (H. R. 1, 2001).
While laudable in principle, the reality was far more complex. A 2009 study conducted by Fordham Law School’s Center on Law and Information Policy found that schools often collected more data than necessary to comply with NCLB and evaluate a school’s overall progress (Reidenberg & Debelak, 2009). In fact, the study noted that state databases included students’ detailed academic, disciplinary, health, and family information, ‘often in a non-anonymous student record’ (Reidenberg & Debelak, 2009). For example, California kept records of students’ parental educational levels, and Iowa and Illinois included data points for students’ criminal histories.
With this mismatch between aspiration and behaviour in the background, parents feared that schools adopting inBloom would end up creating a digital permanent record that tracks, ranks, and categorises students for the rest of their lives (Study session regarding InBloom Inc, 2013). While such a threat was, in itself, ominous, the anxiety was further exacerbated by concern about due process. Parents lacked a clear process for correcting, amending, and erasing incorrect and misleading information contained in the record.
InBloom’s own messaging did little to dissuade fears. Its website listed pages and pages of possible data fields that districts could choose to use (Bulger et al., 2017). While inBloom employees probably perceived this to be a neutral configuration – after all, they were simply listing the fields available without telling districts what specific data should be collected – privacy advocates worried that districts would use all the available fields and collect excessive amounts of data.
The Electronic Privacy Information Center (EPIC) raised concerns that inBloom advertised that it allowed for the creation of ‘principal watch lists’ with no description of why a student might be added to the list. EPIC also criticised inBloom for allowing children to be labelled if they violated ‘norms of behaviour’ (an undefined classification) and categorised as a ‘perpetrator’ or ‘accomplice’ for disciplinary incidents, terms that would normally be used in criminal, not school matters (Study session regarding InBloom Inc, 2013).
Ultimately, inBloom’s leadership erred in their faith that the platform would be seen as sufficiently neutral and that all of the privacy and ethical responsibilities would be transferred to the administrators and teachers at the schools that adopted it. InBloom’s failure to incorporate core Fair Information Principles (another term for the principles we introduced on page 38, like specifying a clear purpose for any data collection and committing to only collect the minimum amount of data needed for that purpose) likely exacerbated privacy concerns.
Public perception was further damaged by inBloom’s privacy policy stating that it could not ‘guarantee the security of the information stored in inBloom or that the information [would] not be intercepted when it [was] being transmitted’ (Ash, 2013). Furthermore, as was the norm for many technology companies, inBloom had a data privacy and security policy that they could unilaterally modify “from time to time, as approved by [an] independent advisory board” (Study session regarding InBloom Inc, 2013). This meant that whatever protections their policy did offer could not be guaranteed; instead, they could change at any time. While these disclaimers and disclosures are standard practice in corporate contracts, this was likely the first time parents had seen these types of clauses. Parents expected that their children’s information would be reasonably protected and expressed unease when it appeared inBloom was dodging responsibility (Ash, 2013).
Finally, and perhaps most importantly, representatives of inBloom did not communicate with parents and advocates effectively (Solove, 2018). An article in ‘Politico’ noted that many educational technology entrepreneurs and school reformers were ‘bewildered by and anxious about the backlash’ against the use of data in education. They had assumed parents would automatically support mining ‘vast quantities of data for insights into what’s working, and what’s not, for individual students and for the education system as a whole,’ and that the benefits were ‘self-evident’ (Simon, 2014). Indeed, the lack of clear answers given to parents and advocates when they raised questions only added to public mistrust and anxiety. Without clear information from inBloom about the platform’s privacy guardrails, parents and advocacy groups narrowly focused on possible abuses of the system.
InBloom lacked a communication plan for addressing the concerns of parents and privacy advocates. It expected school administrators and educators to do the heavy lifting on informing parents about the advantages of the platform. Such overconfidence kept inBloom from providing any communications resources to their education partners. Indeed, they didn’t even inform schools that proactive communication would be necessary. This lack of foresight left schools unprepared to explain how, exactly, the new technology would be beneficial, such as helping parents understand what was happening in their child’s classroom and making it exponentially easier to transfer records when their child transferred schools. Without plausible and positive depictions of how inBloom could responsibly be used, parents had no reason to trust that adopting it would be beneficial. Instead, they had plenty of reasons to be concerned that it would be abused and result in too much information about students being collected. And so, with the bad impressions making a larger impact than the positive ones, every state and district cancelled their contracts with inBloom within one year of its highly anticipated launch (Madda, 2014).
Like inBloom, initiatives around AI in education are often premised on assumptions that ‘everyone’ supposedly agrees with: everyone agrees that data should be easier to share and analyse; everyone agrees that mining data for insights that could help students succeed is worthwhile; everyone wants students to be able to carry their data with them through grades and across districts; everyone wants to take advantage of new technologies to serve students. But if schools or school systems cannot clearly explain how data will be collected, used, shared, and protected, and cannot explain the benefits of initiatives that are based on new data collection or sharing, it will not matter how much that initiative could help students, how much the initiative solves a problem, or whether the initiative has privacy and security built-in. When there is not enough information about an endeavour and privacy and ethics are on the line, people are likely to assume the worst.
Case Study 2 – Mount St. Mary’s
As with the previous case study, this one does not involve the use of AI. Nevertheless, it’s a remarkable cautionary tale for educators considering adopting AI. This is because the fiasco that occurred at Mount St. Mary’s University revolves around predictive analytics, a use of big data that routinely applies AI. Moreover, the problem with how predictive analytics were used at Mount St. Mary’s gets to the heart of a fundamental issue in education itself – equity. The case of Mount St. Mary’s University shines a spotlight on how privacy and ethical guidelines are not only necessary for managing optics, but also for ensuring that the underlying goal of helping each and every student lies at the foundation of all educational initiatives.
Most educators would like to believe that the fundamental goal of education – and associated jobs – is to help every student succeed. It is the guiding ethos of the teaching profession and the true purpose of the work that many view as a vocational calling. However, in the real world, idealism and pragmatism regularly clash. Teaching a diverse student body inevitably requires making compromises and accepting trade-offs. For example, the wellbeing of the majority of students – the students who use the fewest resources and need the fewest interventions – may be prioritised over students with disabilities and students of lower socioeconomic status, who may need more resources and attention. Further complications arise when schools risk losing funding due to lacklustre test scores, low graduation rates and high levels of disciplinary incidents.
While a strictly utilitarian view might justify schools prioritising the interests of the majority instead of trying to apply limited resources equally to everyone, most educators would be repulsed by the prospect of giving up entirely on some children in order to serve the good of the many. If society transitions towards an increasingly data-driven educational model that focuses on maximising efficiency and places increasing priority on AI services, the tension between what is aspirational and what is possible will become more evident.
Mount St. Mary’s University is the second-oldest Catholic university in the United States. In 2014, a private equity CEO and entrepreneur (Svrluga, 2016) was hired as Mount St. Mary’s new president. His goal was to “raise a lot of capital and … start the university on a more aggressive growth trajectory” (Bowie, 2014). With the school board’s approval, he planned to increase the university’s first-to-second year retention rates. Increasing retention rates is significant because the ‘U.S. News and World Report’, an influential publication that ranks colleges and universities, weighs ‘average freshman retention rate’ as one of its highest evaluative categories (Morse, 2013). Schools ranked highly are perceived as prestigious and, as a result, attract quality applicants. Furthermore, alumni can leverage the university’s high profile and ranking when looking for jobs.
During the fall of 2015, Mount St. Mary’s freshmen were asked to take a survey that the president’s office stated would help the school ‘develop better advanced metrics for accepting students’ (Mount President’s attempt, 2016). The survey’s introduction described it as a ‘very valuable tool that will help [students] discover more about themselves’ (Svrluga, 2019). The survey also purported to be ‘based on some of the leading thinking in the area of personal motivation and key factors that determine motivation, success, and happiness’ (Mount President’s Attempt, 2016). Crucially, students were told that ‘there are no wrong answers’ (Svrulga, 2019).
Some questions were innocuous, asking whether students might be interested in certain majors if the school added them. Others were extremely sensitive, asking whether the student had a learning disability, whether a close friend or family member had died in the past year, or whether the student felt they ‘could not shake off the blues, even with help from … family and friends’ (Svulga, 2019).
Unbeknownst to the students and most faculty members, Mount St. Mary’s president planned to use the survey results to inflate the school’s retention rates by asking select students to leave the university (Schisler & Golden, 2016). The administration would contact some of the students whose survey results labelled them at risk of leaving the university and offer them a tuition refund if they dropped out before the federal reporting deadline for the school’s first-year enrolment. When some faculty protested, the president stated, “[t]his is hard for you because you think of the students as cuddly bunnies, but you can’t. You just have to drown the bunnies … put a Glock to their heads” (Schisler & Golden, 2016).
Despite his confidence, the plan failed. The faculty refused to identify the quota of twenty to twenty five students to talk into dropping out, the student newspaper reported on the scheme, and, eventually, enough outrage resulted that the president resigned (Johnson, 2017).
However, he continued to maintain that he was doing the right thing for both the college and students who were likely to fail. According to an op-ed he wrote in ‘The Washington Post’:
‘[r]ather than continuing to collect exorbitant sums of money from the families of continually failing students, the kindest, most responsible option for institutions may be to return students their paid-in tuition and offer guidance for other paths [such as] other universities or technical schooling . . . many students aren’t always willing to raise their hand and say, “I need help.” So, it is our obligation to identify warning signs that can appear as early as a student’s first semester that the academics and college life is not the right fit’ (Svrluga, 2017).
But a sociological analysis of this case found that the survey questions lacked an ‘established connection to educational settings’ and, further, lacked ‘evidence of any effort by the university to test validity or reliability’ to ‘measure of the likelihood of academic success’ (Johnson, 2017). The questions focused largely on ‘non-cognitive characteristics of students’, which included sections on ‘resilience and grit, personality inventories...religious beliefs’ and even included a section for evaluating students for clinical depression (Johnson, 2017).
Another problem with this calculus – beyond the lack of scientific rigour underlying the survey and prior statements where he had expressed anything but sympathy for struggling students – is that the underlying assumptions were not predicated upon universally shared assumptions. While his choice was to push students who seem to have a higher likelihood of failure out of the university, there were other choices, including providing students with more support to prevent them from failing. One first-generation Mount St. Mary’s student interviewed after the president resigned described her first year as “a struggle for me, it was traumatic … [e]very day I would call my mom, sobbing, ‘I don’t think I can do this.’” Fortunately, with a faculty advisor’s help, she persisted, and was a successful third-year student at the time of the interview. “If somebody would have told me when I was a freshman, ‘this is not a good fit for you, you should probably go,’ I would have gone” (Scott, 2019).
No matter how much information a school has about students, and no matter how smart a human or machine’s analysis of it becomes, it is impossible to predict with 100% certainty who will decide to drop out of college. As society aims to create more educational opportunities for lower income and minority students, we should not lose sight of the fact that, since fewer students like them have historically succeeded, predictive algorithms trained on historical data will label these students less likely to succeed here and now. Imagine how such analytics would have rated the chances of women graduating from medical school when universities first began admitting women.
Beyond the potential for discrimination, automated decision-making processes like predictive analytics are not neutral. They are imbued with the values of their creators. In the case of Mount St. Mary’s, the administration’s values were not aligned with faculty or students. The survey’s wording led students to believe there were no wrong answers, encouraging them to answer truthfully and giving them the impression that their answers would not be used against them.
Consequently, students who took the survey consented to the use of their personal information for the survey’s stated purpose – improving the school and student experience – but not its actual purpose – culling the student body to meet the president’s goals for the university. Not only does this case study illustrate what happens when an administration fails to be transparent with data collection and analysis, but it also illustrates the importance of fairness. Students are the largest stakeholders in the context of student privacy conversations. They deserve complete honesty when it comes to why their data is being collected and how it will be used.
Imagine another universe where Mount St. Mary’s created the exact same survey, but instead of pressuring students to leave, they presented them with opportunities for more guidance counselling, tutoring, or a re-ordered class schedule designed to improve student success. If the administration had adhered to privacy and ethical guardrails – such as maintaining a primary focus on the best interests of each student, data minimisation, equity, and transparency – it is unlikely that this initiative would have become a case study in how predictive analytics could harm students.
Teaching AI and privacy ethics
In the previous sections, we highlighted many interrelated ethical and privacy risks associated with big data and AI. We’ll expand the discussion here and offer suggestions for how instructors can effectively teach some of the topics.
As with all subjects, teachers should design age-appropriate lessons. Until recently, it was nearly impossible to find educational material on AI ethics that weren’t created for university students or professionals. Fortunately, things are starting to change. Blakely Payne, a graduate student at the Personal Robotics Group at the MIT Media Lab, created an important resource that instructors at various levels can use and modify. It’s an open-source, module-based curriculum for middle school students on AI that can be integrated into science and humanities classes. Access the material at MIT Media Lab.
Engaging lessons that combine technical and ethical content
Payne’s curriculum is important for three reasons. First, it covers both technical and ethical concepts. Second, it provides examples that students will find resonant. Third, it encourages students to view AI systems as changeable. By combining technical and ethical education, Payne’s approach provides a model for instructors at all levels to emulate. Including technical information is valuable because it helps students develop the technical literacy needed to understand AI.
For example, students begin by learning the basics – understanding what algorithms are. Payne recognises that a useful entry point is for students to view algorithms as recipes containing instructions for generating outputs after acting upon inputs in designated ways. To make this point in an engaging manner, Payne includes an exercise for kids to brainstorm which instructions an algorithm should follow to make the ‘best’ peanut butter and jelly sandwich. Since the sandwich only has a few ingredients, students might presume it’s a basic task. However, in short order, they’ll realise it’s actually a surprisingly complicated endeavour. Moreover, with help from instructors, students can begin to appreciate that the complexity in this seemingly mundane case actually speaks to deep issues that lie at the heart of many consequential applications of AI.
Making the best peanut butter and jelly sandwich isn’t an endeavour that can be reduced to automating a basic process because what ‘best’ means is undefined. Is it an aesthetic ideal, guiding the algorithm to make the best tasting or the best-looking sandwich? Or should ‘best’ be understood as an ideal about safety, guiding the algorithm to make a sandwich that tastes like peanut butter and jelly, but that kids with peanut allergies can eat without getting sick? By helping students appreciate that the definition of concepts like ‘best’ are rooted in ethical values with diverse interpretations, they can be guided towards the eureka moment of grasping that programming algorithms inherently comes with the risk of, to continue with the culinary metaphor, baking in questionable preferences and unfair biases. Becoming aware of this risk – which fundamentally links technical and ethical components of AI – is a teachable skill that enhances the broader skillset of ethical attunement, which educators at every grade level should aspire to develop in their students.
Instructors can cover more advanced material by modifying this engaging approach combining technical and ethical lessons. For example, consider the ethical issue of fairness in the context of AI. As we have already noted, the ideal of fairness is mentioned in the OECD privacy guidelines. However, the concept of fairness, like the concept of best, has additional definitions that correspond to a range of ideals. Fairness encompasses everything from equality of opportunity in situations where candidates are applying for jobs and AI plays a role in determining who is and isn’t hired, to equality in outcome in cases where AI helps determine who gets pulled over for questioning during airport security checks (Binns, 2018).
In an ambitious study of prominent AI ethics guidelines developed around the world (including a discussion paper from the Australian Government Department of Industry, Science, Energy and Resources) Anna Jobin, Marcello Lenca, and Effy Vayena discovered a ‘global convergence’ around five ethical principles: ‘transparency, justice, and fairness, non-maleficence, responsibility and privacy’ (Jobin et al., 2019). (Note: The fact that privacy is listed as one of the most fundamental AI ethics issues corroborates the approach we have been taking here of treating ethics and privacy as deeply connected.) The authors succinctly summarise key findings of an extensive literature review that traverses public and private sectors, carefully explain what the most important AI ethics concepts are and why they matter, and identify some of the most important commonalities and differences in how ethical principles are interpreted.
Let’s bring the discussion of fairness back to teachable examples. The controversy surrounding the use of the U.S. Correctional Offender Management Profiling for Alternative Sanctions (COMPAS) system is an example illustrating the difficulty of translating diverse ethical interpretations of fairness into mathematical models. COMPAS, developed by the private company Northpointe, is a recidivism risk assessment system that predicts the likelihood a defendant will re-offend in the future. U.S. judges use COMPAS as an aid in conjunction with other information when deciding whether or not a defendant should be confined to jail or released on bail until the criminal trial takes place. The hope was that the system and others like it could improve justice by helping generate fairer predictions.
COMPAS generates its results from a questionnaire on the defendant’s criminal history and attitudes about crime, explicitly not including race as a variable for analysis. However, investigative reporting by ProPublica found that COMPAS was twice as likely to erroneously flag black defendants as being high-risk for committing future crimes, with the opposite holding for white defendants, who were twice as likely to be flagged as low-risk (Angwin et al., 2016). The higher risk scores generated by COMPAS do, in fact, correlate with the likelihood that someone will be rearrested, and is nearly identical across racial lines – a result that, taken on its own and without regard for broader context, appears fair because predictions about future criminal offences should apply equally to everyone in a risk category regardless of their race. So, why is it that COMPAS is harsher on black defendants? It appears to be because in the U.S. black defendants have historically been rearrested at higher rates than white ones, and proxies for race, such as prior arrests, can lead COMPAS to classify black defendants as higher risks than white defendants (Corbett-Davies et al., 2016). Although COMPAS was designed to analyse risk without racial bias, historical prejudice nevertheless contributed to biased predictions.
It can be more difficult for students to grasp the idea that different, sometimes conflicting, conceptions of fairness exist than appreciating that there are different ways to make the best peanut butter and jelly sandwich. The danger of algorithms using data tainted by histories of injustice, such as de-contextualised statistics that make minorities seem like criminals or poor job candidates, makes issues surrounding AI and fairness especially timely. Modelling educational exercises that follow Payne’s engaging model of combining technical and ethical lessons will help educators effectively communicate both concepts to students. For example, Karen Hao and Jonathan Stray created ‘Can you make AI fairer than a judge?’ for the ‘MIT Technology Review’. It is an interactive online article that explains the main issues in the COMPAS example in an engaging way, encouraging readers to play a courtroom algorithmic game where they explore issues like trying to make the fairest possible risk-prediction algorithm (Hao & Stray, 2019).
Lessons that focus on examples students can relate to
Payne is right to realise that K-12 educators should primarily focus on examples that will resonate with students based on their personal experiences and professional aspirations. Most conversations about AI focus on potential existential risks set in the distant future with technology that does not yet and may never exist.
Payne focuses on relatable examples because she recognises that children are growing up in a digital world increasingly mediated by AI. Algorithms suggest what they should watch, read and listen to. Since kids are a vulnerable demographic, and the technologies they use in and outside of the classroom will shape everything from what they know to who they are and aspire to be, it’s absolutely critical they are empowered to use them wisely and be critical consumers. To this end, Payne includes a module on redesigning YouTube, a platform recently criticised for nudging kids towards dangerous, conspiratorial content (Roose, 2019).
Her lesson plan has students identify the various stakeholders that YouTube impacts and construct an ethical matrix that highlights where their values overlap and conflict. This exercise is ideal for learning how prioritising different stakeholders with different preferences and values can lead to designing recommendation algorithms that perform in different ways. Crucially, this analytic and pedagogical approach can be usefully applied and adapted to cover a range of AI systems that students should be expected to care about.
We expect students will be interested in how AI is used in schools since these applications can directly impact their lives and futures. This makes examples like the Mount St. Mary’s case especially relevant. The scenario can be adapted to K-12 schools considering using AI to predict who will and won’t benefit from participating in a special program.
Students could write an essay that imagines the school administrators proposing the program to an AI ethics board. Students could pretend to be ethics board members, and writing from this perspective, they could determine who the relevant stakeholders are and what values they embody. Specifically, they should decide to approve or reject the proposal based upon considerations related to them, as well as the OECD principles and AI ethics principles that Jobin, Lenca, and Vayena cover.
An important issue for students to grapple with in this context is what definition of fairness is implicated. We already alluded to it in our previous discussion – it’s the problem of statistical discrimination that fails to treat people as individual decision-makers who might or might not behave like other members of the groups they are identified as belonging to (Binns, 2018).
Similarly, we also expect students will be interested in topics that concern how AI will be used to impact the lives of their parents and other family members. Thus, teachers might want to design assignments that have students think carefully about examples like the Australian controversy surrounding Robodebt that we discussed earlier. Students could reflect on how they would feel if their parents were incorrectly flagged by such a system, and how they would design a better system to prevent the injustice from occurring.
Training tomorrow’s responsible leaders today
Since today’s children are tomorrow’s leaders, it’s important to expose them to the profound ethical and privacy consequences of AI as early as possible. This way, as they get older and start working on projects involving AI, they’ll hopefully be inclined to care about ethical issues from the very start of a project. This ethically attuned outlook is preferable to seeing ethics as an afterthought. Making ethically important changes after a project is completed can be burdened by undue expenses or difficulties that could have been avoided through better planning. The ethically attuned outlook is also preferable to passing responsibility for addressing ethical concerns to other bodies, like regulators. Forgoing this responsibility implicitly rests on the outdated and fallacious presumption that scientists and engineers are not at all responsible for how their devices, systems and ideas are applied (Green, 2018).
We believe it is especially important, as Payne declares that “the ultimate goal is to enable students to see artificial intelligence as manipulatable – from a technical and societal standpoint – and to empower students with tools to design AI with ethics in mind.” Pushing this point further, we would like to suggest that students be given the opportunity to consider cases where the most ethical outcome might be to prevent AI from being used in the first place.
Consider the example we previously discussed of students objecting to being required to learn by using a face scanning engagement system. As mentioned, the CEO of a company that makes one of these systems dismissed their concerns as irrelevant because they are out of step with a presumably inevitable technological evolution. In the classroom, students could be given an opportunity to articulate precise objections to an AI-informed product, like the engagement scanner, and brainstorm ways that the product could be changed or policies could be put in place to promote quality education while mitigating against student concerns. Students should also be given the chance to explain if and why changes or policies won’t suffice and how educational goals could be better met without a particular form of AI or possibly without using AI at all.
By helping students consider the full range of possibilities, educators can truly prepare students to meet the AI imperative – an imperative that does not require solving every possible problem with AI.
Acknowledgements
The authors would like to thank the following individuals for their help and support with this article: Sara Collins, Brenda Leong, Jasmine Park, Anisha Reddy, Alexis Shore, and Katherine Sledge.
References
Angwin, J., Larson, J., Kirchner, L., & Mattu, S. (2016, May 23). Machine bias. ProPublica. https://www.propublica. org/article/machine-bias-risk-assessments-in-criminal- sentencing.
Arizona State University. (2018). Connecting the dots at ASU. https://fullcircle.asu.edu/external/connecting-the- dots-at-asu/
Ash, K. (2013, April 16). InBloom aims to increase data flow despite controversy. Education Week. http://blogs. edweek.org/edweek/DigitalEducation/2013/04/inbloom_ aims_to_increase_data_.html
Binns, R. (2018). Fairness in machine learning: Lessons from political philosophy. Proceedings of machine learning research 81, 1-11. http://proceedings.mlr.press/ v81/binns18a/binns18a.pdf
Bowie, L. (2019, May 28) Simon Newman named Mount St. Mary's president. Baltimore Sun. https://www. baltimoresun.com/education/bs-md-mount-st-marys- president-20141208-story.html.
Bulger, M., McCormick, P., & Pitcan, M. (2017). The Legacy of InBloom. Data & Society. https://datasociety.net/pubs/ ecl/InBloom_feb_2017.pdf
Clark, P., Etzioni, O., Khashabi, D., Khot, T., Mishra, B. D., Richardson, K., Sabharwal, A., Schoenick, C., Tafjord, O., Tandon, N., Bhakthavatsalam, S., Groeneveld, D., Guerquin, M., & Schmitz, M. (2019). From ‘F’ to ‘A’ on the N.Y. Regents Science exams: an overview of the Aristo project. Allen Institute for Artificial Intelligence. https:// arxiv.org/pdf/1909.01958.pdf
Corbett-Davies, S., Pierson, E., Feller, A., & Goel, S. (2016, October 17). A computer program used for bail and sentencing decisions was labeled biased against Blacks. It's actually not that clear. The Washington Post. https:// www.washingtonpost.com/news/monkey-cage/ wp/2016/10/17/can-an-algorithm-be-racist-our-analysis-is- more-cautious-than-propublicas/
Dean, L. (2019, November 19). Government announces major robodebt changes. Yahoo! Finance. https:// au.finance.yahoo.com/news/robodebt-shake-up-as- government-backs-down-042716284.html.
Denver Post. (2016, April 29). InBloom enables great teaching tools. The Denver Post. https://www.denverpost. com/2013/07/18/inbloom-enables-great-teaching-tools/
Eveleth, R. (2018, October 29). Facing tomorrow's high- tech school surveillance. Vice. https://www.vice.com/ amp/en_us/article/j53ba3/facial-recognition-school- surveillance-v25n3.
Emerging technology from the arXiv. (2013, October 3) The big data conundrum: how to define it? MIT Technology Review. https://www.technologyreview.com/s/519851/the-big-data-conundrum-how-to-define- it/
Family Online Safety Institute. (2019). Online safety in the age of artificial intelligence. https://www.fosi.org/policy- research/online-safety-artificial-intelligence/
Future of Privacy Forum. (n.d.). Ethics, governance, and compliance resources. https://sites.google.com/fpf.org/ futureofprivacyforumresources/ethics-governance-and- compliance-resources?authuser=1
Gellman, R. (2019). Fair Information Practices: A Basic History. https://bobgellman.com/rg-docs/rg-FIPshistory. pdf
Green, Ben. (2018). Data science as political action: grounding data science in a politics of justice. arXiv preprint. https://arxiv.org/abs/1811.03435
Greenleaf, G., Clarke, R., & Waters, N. (2013). International Data Privacy Standards: A Global Approach (Australian Privacy Foundation Policy Statement). UNSW Law Research Paper 2013(62). https://papers.ssrn.com/sol3/ papers.cfm?abstract_id=2327325
Hao, K., & Stray, J. (2019, October 17). Can you make AI fairer than a judge? Play our courtroom algorithm game. MIT Technology Review. https://www.technologyreview. com/2019/10/17/75285/ai-fairer-than-judge-criminal-risk- assessment-algorithm/
Haw, M. (2019, September 6). Will AI replace university lecturers? Not if we make it clear why humans matter. The Guardian. https://www.theguardian.com/ education/2019/sep/06/will-ai-replace-university-lecturers-not-if-we-make-it-clear-why-humans-matter.
Jobin, A., Marcello I., & Vayena, E. (2019). The global landscape of AI ethics guidelines. Nature Machine Intelligence, 1, 389–99. https://doi.org/10.1038/s42256-019-0088-2
Johnson, J. (2017, February 24). Structural justice in student analytics, or, the silence of the bunnies [Paper presentation]. Digital sociology mini-conference: Eastern Sociological Society annual meeting, Philadelphia. https://the-other-jeff.com/wp-content/ uploads/2017/02/Structural-Justice-in-Learning- Analytics-ESS.pdf
Karp, P. (2019, November 19). Robodebt: government abandons key part of debt recovery scheme in major overhaul. The Guardian. https://www.theguardian.com/ australia-news/2019/nov/19/robodebt-government- abandons-key-part-of-debt-recovery-scheme-in-major- overhaul
Madda, M. (2014, April 21). What InBloom's Shutdown Means for the Industry. EdSurge. https://www.edsurge. com/news/2014-04-21-what-inbloom-s-shutdown- means-for-the-industry
Marcus, G. (2019, November 30). An Epidemic of AI Misinformation. The Gradient. https://thegradient.pub/ an-epidemic-of-ai-misinformation/
Marsh, S. (2019, November 19). Federal Government immediately halts part of Centrelink robodebt scheme. 9News. https://www.9news.com.au/national/centrelink- robodebt-to-be-halted-immediately-debts-frozen/ c9e2dc06-efda-4e58-a9d2-cdd15a43c856
McCarthy, J. (2007). What is artificial intelligence? Stanford University. http://www-formal.stanford.edu/jmc/ whatisai/
Morse, R. (2013, September 9). Best colleges ranking criteria and weights. US News & World Report. https:// web.archive.org/web/20140802233928/http://www. usnews.com/education/best-colleges/articles/2013/09/09/ best-colleges-ranking-criteria-and-weights
Mount President's attempt to improve retention rate included seeking dismissal of 20-25 first-year students. (2016, January 19. FIRE. https://www.thefire.org/mount- presidents-attempt-to-improve-retention-rate-included- seeking-dismissal-of-20-25-first-year-students/
No Child Left Behind Act 2001, H. R. 1, 107th Cong,., 1st Sess (2001).
Sainato, M. (2015, August 19). Stephen Hawking, Elon Musk, and Bill Gates warn about artificial intelligence. Observer. https://observer.com/2015/08/stephen- hawking-elon-musk-and-bill-gates-warn-about-artificial- intelligence/
Schisler, R, & Golden, R. (2016, January 19). Mount President’s attempt to improve retention rate included seeking dismissal of 20-25 first-year students. The Mountain Echo. http://msmecho.com/2016/01/19/mount- presidents-attempt-to-improve-retention-rate-included- seeking-dismissal-of-20-25-first-year-students/
Scott, A. (2019, April 29). Mount St. Mary's president resigns in the wake of ‘bunnies’ comment. Marketplace. https://www.marketplace.org/2016/02/29/ mount-st-marys-u-quest-better-numbers/
Selinger, E. (2019, October 14). Why technologists fail to think of moderation as a virtue and other stories about AI. Los Angeles Review of Books. https://lareviewofbooks. org/article/why-technologists-fail-to-think-of- moderation-as-a-virtue-and-other-stories-about-ai/
Simon, S. (2014, June 8). Big brother: meet the parents. Politico. http://www.politico.com/story/2014/06/internet- data-mining-children-107461.html
Solove, D. (2018, November 30). Why did inBloom die? A hard lesson about education privacy.
TeachPrivacy, https://teachprivacy.com/inbloom-die- hard-lesson-education-privacy/
Svlurga, S. (2016, January 19). University president allegedly says struggling freshmen are bunnies that should be drowned. The Washington Post. https://www. washingtonpost.com/news/grade-point/wp/2016/01/19/ university-president-allegedly-says-struggling- freshmen-are-bunnies-that-should-be-drowned-that-a- glock-should-be-put-to-their-heads
Svrluga, S. (2019, April 29). The freshman survey that rang alarm bells for some at Mount St. Mary's. The Washington Post. https://www.washingtonpost.com/ news/grade-point/wp/2016/02/12/the-freshman-survey- that-rang-alarm-bells-for-some-at-mount-st-marys/
Study session regarding InBloom, Inc. Colorado State Board of Education (2013) (testimony of Khaliah Barnes).
Reidenberg, J., & Debelak, J. (2009). Children's Educational Records and Privacy. Fordham Center on Law and Information Policy. https://www.fordham. edu/info/23830/research/5922/childrens_educational_ records_and_privacy
Roose, K. (2019, February 19). YouTube unleashed a conspiracy theory boom. Can it be contained? The New York Times. https://www.nytimes.com/2019/02/19/ technology/youtube-conspiracy-stars.html
Introduction
In 1956 computer scientist John McCarthy coined the phrase ‘artificial intelligence’ (AI) to describe ‘the science and engineering of making intelligent machines’ (McCarthy, 2007). Over time, the term has evolved to cover a variety of technologies, including ones widely used in education, from plagiarism detectors to voice-activated virtual assistants leveraged to enhance campus information distribution and classroom pedagogy (Arizona State University, 2018).
Contemporary AI discussions are about ‘a variety of methods and tools which mimic cognitive functions across three areas: 1) perception/ vision, 2) speech/language, and 3) learning analysis’ (Family Online Safety Institute, 2019). Experts further distinguish between ‘narrow AI’ and ‘artificial general intelligence’. Narrow AI performs well in discrete tasks – think of algorithms that are good at playing chess but wouldn’t help someone drive a car, and vice versa – and is currently having a big impact on society. By contrast, artificial general intelligence can learn across domains and think for itself, possibly in astonishingly more sophisticated ways than humans do. For now, artificial general intelligence is the stuff of science fiction and unresolved debates rage over whether it will ever be created.
Presently, the advancement of AI is associated with machine learning and its subset deep learning. Machine learning involves algorithms using statistics to computationally identify patterns in data sets that are often so large they are referred to as big data (Emerging technology from the arXiv, 2013). Big data is the foundation on which modern AI is built. This vast quantity of data gives scientists and statisticians more opportunities to create AI that can identify increasingly intricate and nuanced patterns. Since these patterns are frequently about how humans think and act, they can be used to make sense of present human behaviour. For example, AI can analyse student facial expressions to infer whether students are bored or engaged while doing an assignment. AI can also be used to more accurately predict future human behaviour. For example, it might be used to estimate which students will be academically successful and which candidates will perform best at a job by scanning for certain keywords in a resume that match or are similar to the resumes of current successful accepted students or employees when they were initially hired. Without big data, these AI applications would not exist. In this article, we use the term AI to refer not only to the definition provided in the previous paragraph, but also to the infrastructure that is used to create the AI, which includes big data, statistical modelling, and machine learning.
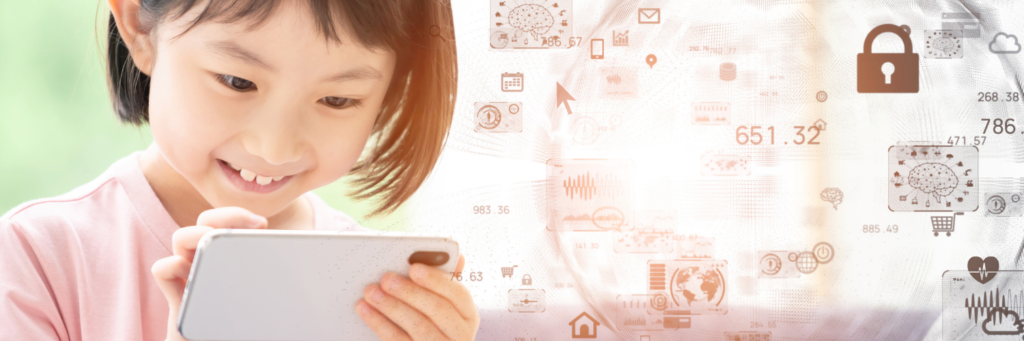
Because this article focuses on privacy and ethics issues in K-12 education, we won’t get into the specific details of technical discussions about AI. Instead, our goal is to identify practical educational uses of tools and systems associated with AI and big data. We will offer clear principles for responsibly using AI and its related big data in educational settings, and make recommendations for how instructors can effectively teach students about the ethical and privacy risks associated with AI and big data in and beyond the educational context.
Although we won’t review any products, from the start we would caution educators and administrators to use care when selecting technologies that are marketed as powered by AI. As discussed earlier, the term AI can encompass a variety of technologies and be interpreted in many ways. Journalistic coverage of AI products and services has been riddled with misinformation. Consequently, companies have enough wiggle room to manipulatively peddle their wares and abuse the term to create exaggerated impressions of how cutting-edge their products are (Marcus, 2019).
How can educators meet the AI imperative?
To put it mildly, high hopes exist for AI. Beyond widely-covered victories of AI over human champions in games requiring immense knowledge, skill, and creativity, such as IBM Watson in Jeopardy!, Google AlphaGo in Go, and AlphaZero AI in chess, AI is being adopted in a range of domains, from medical to military applications to the educational sector. One AI-powered educational testing system is so advanced that it scored over 90% on the multiple-choice questions found on the eighth grade standardised science exam administered in New York State (Clark et al., 2019).
Experts believe AI will significantly impact how teachers instruct, how students engage with learning, and how administrators set priorities and select policies. Consider the following thought experiment about an advanced AI tutor. The scenario has a few distinctive features: it takes place in the future where technological capabilities are deployed that don’t yet exist, it envisions university students learning in virtual classrooms and, crucially, it represents what some in the education technology sector consider a positive step forward.
“The AI tutor will design personalised learning plans that optimise each student’s outcome. Should one student watch their lecture at breakfast time, or in the evening? Where should their first test pop up in a busy schedule? How much preparation will they need to understand a certain concept? While a skeleton crew of humans would be needed initially to design curriculums (the creative bit) and film lectures (CGI is still too expensive), AI tutors could do the rest” (Haw, 2019).
Regardless of debates about whether the scenario described above is desirable or advantageous for students, the most important pedagogical tasks related to AI don’t simply keep humans in the loop, but at the centre of the action. Educators must figure out how to best ensure students are prepared to succeed in a world where AI is deeply embedded in infrastructure and its use influences what jobs are available, what civic life is like, how markets function, and how people communicate and socialise.
Simply put, 21st century education requires preparing students for an AI-intensive future. Educators in primary and secondary schools need to create new lessons and experiment with new educational technologies that can help them meet the challenge of the AI imperative.
The AI imperative is the requirement that educators adequately understand what AI is and the key privacy and ethical issues surrounding its use, effectively teach grade-appropriate versions of that knowledge, and put AI tools to good use in and around the classroom. Failure to meet the AI imperative will leave students unprepared for the increasingly AI-reliant real world. With the stakes so high, the pressure to meet the AI imperative eventually will come from everywhere: from parents, politicians, employers, technology companies, and even the students themselves.
What does it take to meet the AI imperative? Clearly, technical proficiency is essential. For example, educators will need to teach students what algorithms are and gain experience creating programs that involve algorithmic problem-solving and computational thinking. However, this is not enough: without due consideration of privacy and ethics, world-class computer science lessons on machine learning and other forms of AI will fall short. Technology companies themselves acknowledge that this is the case by publishing company-backed ‘AI principles’ that outline vision statements infused with ethical values and commitments (Future of Privacy Forum, n. d.). Research institutions and public sector organisations also recognise the importance of privacy and ethics by proposing principles and guidelines for ethical AI applications (Jobin et al., 2019).
These formal initiatives are also driven by headline-grabbing controversies that have raised the public’s consciousness about the importance of algorithmic accountability. For example, the Australian Online Compliance Intervention program, colloquially referred to as Robodebt, found inconsistencies between Australian Taxation Office income and welfare payments. The program was deployed to generate debt notices and secure ‘up to $4.5 million in Centrelink debt a day’ (Dean, 2019). After, after ‘tens of thousands of welfare recipients’ claimed they were ‘overcharged for alleged debts,’ the federal government decided to change the program. As initially implemented, the program left the burden on wrongly targeted recipients to prove they were treated unfairly (Karp, 2019).
Now, human officers are no longer permitted to exclusively rely on the automated system and are required to conduct ‘further investigations to determine whether a debt exists’ (Marsh, 2019). Mandating human oversight over important decisions is a recurring concern expressed in debates over AI accountability.
Without proper human oversight, education-based AI initiatives can also result in privacy and ethical violations. Respecting student privacy should be a critical component of any effort to meet the AI imperative. Protecting student privacy is not only the right thing to do – it can be legally required. And, because promising programs can be destroyed by poor privacy optics, respecting privacy is instrumentally valuable. If schools or schooling systems communicate poorly with parents about the privacy precautions they are taking and the privacy policies they are following, they may shoulder some of the responsibility if adverse outcomes follow. Opportunities that are genuinely good for students can be misconstrued as dangerous and get shut down if judged irresponsible.
Meeting the AI imperative also requires educators to teach students about the ethical risks of big data and AI systems. Without this knowledge, students won’t be prepared to understand and navigate an increasingly AI-centric world. Quality approaches to teaching ethical risks, including privacy pitfalls, require educators to practice what they preach. If students and parents believe that educators are not modelling good behaviour, their authority could be undermined.
A key lesson that educators should stress is that the most basic way AI will change society is through the choices people make about which AI systems to adopt and reject, and how to wisely use the ones that are selected. By acting as if the AI imperative requires fatalistically acquiescing to a contingently popular technological trend, people neglect their agency and abdicate basic responsibility.
It is simple for educators to rationalise away accountability by acting as if a technologically determined pathway exists, mandating how students must learn. For example, a group of students expressed discomfort about being required to use a classroom engagement detection system that scans students’ faces and uses AI to infer whether they are engaging with material in an attentive manner. When asked to comment on this, the CEO of the company that produced the system bluntly replied that the students’ concerns would not change anything; channelling Darwin, he noted “[e]verybody is doing this. It's really early and shocking, but we cannot go against natural laws of evolution” (Eveleth, 2018).
What a striking response! Starting with the age-old question, “Would you jump off a bridge just because everyone else is doing it?”, we teach young children that something is not necessarily right just because other people are doing it. But adults may fail to heed their own advice when the AI imperative seems to only provide two options: accommodate and adapt all potential applications of AI or perish and give up hope of being relevant.
Technological trends are not natural laws that force individuals and institutions to go in any particular direction. When people, including students, articulate justified ethical reservations that push back against popular positions, seriously considering their concerns can help us forge a better future. Educators should not lose sight of this fact by acting as if they are powerless to contribute to decisions about how to go about meeting the AI imperative.
In the case of the automated engagement detection systems, it is wrong to automatically dismiss student concerns. Without seriously considering the merit of their criticisms, it is impossible to identify students’ values, whether the values deserve the attention of educators and administrators, and whether greater emphasis on these values can motivate the educators using these systems to meet their pedagogical goals through better alternatives.
Students who are dismayed about AI surveillance and analysis in the classroom are often mirroring broader concerns in societal controversies about policing and workplace dynamics. Giving concerned students the impression that advocating for privacy is passé and that authorities should be given unrestricted power over their lives diminishes student agency and threatens their autonomy.
Ingrained privacy and ethics guardrails
We’ve already provided some of the reasons why student privacy should be respected. But let’s think about this issue on a more fundamental level. Clearly, students aren’t entitled to absolute privacy. For safety reasons, teachers and administrators might be justified in searching a locker or a student’s bag for drugs or responding to threats made over social media. If disciplinary problems arise in schools, educators can be obligated to contact parents rather than keep the information confidential. Nevertheless, there are many reasons why students deserve to have some privacy interests protected.
Students are a vulnerable population. Consequently, lack of adequate privacy safeguards can lead to students experiencing embarrassment and shame, even trauma, and suffering lasting reputational damage that can unfairly damage or diminish their future opportunities. Insufficient privacy protections also can lead to exploitation; that’s why many believe that technology companies providing educational services should be prevented from benefitting in any way from student data, even from using it to improve their products. Inadequate privacy measures can also have a chilling effect, inhibiting student self-expression. This harm can impede learning because students who are anxious about privacy issues in schools may have difficulty focusing on scholastic tasks, like lectures and exams. In turn, this can compromise their grades and intellectual maturation. Furthermore, the chilling effect can compromise students’ ability to socialise.
Socialisation is critical to the healthy development of students that schools are supposed to foster. Since schools are responsible for preparing students to become citizens, schools should strive to empower them to think critically about when it is appropriate or inappropriate for authorities, like government officials, employers, and technology companies to engage in privacy diminishing activities like surveillance. If students and parents believe that schools are not adequately protecting their privacy, they might intervene in ways that compromise, if not shut down entirely, promising educational programs.
Since schools should teach students how to grow into responsible, autonomous adults, they should avoid smothering forms of monitoring that lead students to abdicate responsibility for their own behaviour as well as avoid forms of surveillance that will inhibit students from full participation in educational activities. Ideally, schools will provide students with tools for having thoughtful conversations and making thoughtful decisions about privacy in both analogue and digital spaces with their parents, teachers and peers.
For the reasons just provided, it is difficult to neatly distinguish between privacy and ethics. Many privacy protections are rooted in ethical ideals, like respecting autonomy and fostering personal and social development. A useful way to think about the relationship between privacy and ethics is to revisit what happened when data digitisation began in the 1970s. At that time, government officials and policymakers expressed concern, often ethically-minded, about the implications the innovation would have on privacy. Worldwide, committees and commissions were formed to determine the rules for public and private entities that collect, process and share computerised data.
These efforts culminated in the Organisation of Economic Cooperation and Development (OECD) releasing the ‘OECD Guidelines on the Protection of Privacy and Transborder Flows of Person Data’ in 1980, drafted by a group chaired by the then-Chairman of the Australian Law Reform Commission (Gellman, 2019). The guidelines included eight core ideas (paraphrased below) that now lie at the foundation of many privacy laws and proposals around the world. Australia adopted them in 1984, and today these established principles can help provide privacy and ethical guardrails for K-12 institutions to responsibly adopt AI (Greenleaf et al., 2013).
- Information collection should be It should be collected in a fair way, ideally with the knowledge or consent of the person whose data it is.
- Information needs to be accurate, complete, and up-to-date.
- Information should only be collected for a specific purpose that is clearly specified before collection The information should not be reused later in ways that are incompatible with the original specific purpose.
- Information should not be shared or reused in ways incompatible with the original specific purpose without either consent of the person whose data it is or a law providing the authority to do so.
- Information should be protected through reasonable security safeguards that limit the risk of an unauthorised person accessing, using, changing, sharing, or destroying that information.
- There should be transparency about how information is collected, used, shared and protected.
- People have certain rights about their own information, including the right to know what information others have about them, who has it, and the right to request that information be corrected, amended or erased.
- Those holding data must be held accountable for the above principles.
To see how these principles matter in practice, we offer two case studies where privacy and ethical missteps compromised potentially positive advancements in data sharing and educational technology.
Case Study 1 – inBloom
In Australia, inBloom might not be a well-known initiative. However, its rise and fall fundamentally shaped the educational technology landscape in the United States. Even though it is not a cautionary tale about the use of AI, the manner in which the debacle unfolded holds significant lessons for educators everywhere who want to responsibly use and communicate about new educational technologies, particularly in contexts where big data is involved. Indeed, cases like inBloom are especially important to consider now in the early days of using AI in education. Some of the clearest lessons about privacy and ethics can be learned by critically analysing predecessor cases that raise similar issues.
InBloom was a $100 million non-profit educational technology initiative that ran from 2013 to 2014 and aimed to improve American schools by providing a centralised platform for data sharing, learning applications and curricula (Bulger et al., 2017). At the time, many education reformers and student advocates argued that education data should be democratised, and that students should be able to ‘carry’ their data with them from grade-to-grade and post-graduation in a ‘digital backpack’. Despite lofty ambitions, scholastic digital data systems adopted in the 1990s and 2000s were clunky (Denver Post, 2016). Sometimes, data was not even transferable between schools in the same state. Moreover, the systems were often built around minimally useful data points that were used to evaluate students and educators at a single moment in time, instead of continually throughout the year, such as grades and test scores rather than digital portfolios.
The data inBloom could store and the technical architecture of the software were portrayed as game-changers. InBloom was developed to provide secure, semi-instantaneous access to student information, able to facilitate personalised learning, identify learning issues before they manifested in failing grades, provide students and their parents easy access to their own continually updated data, and, overall, help create a more data-driven educational system. Many of these objectives underlie the current push for schools to take advantage of big data and AI.
Soon after inBloom launched, it secured contracts with several states and school districts (Bulger et al., 2017). For many parents, inBloom’s launch was the first time they heard about how data collection and use in the educational sector had changed since the passage of the major U.S. education law, No Child Left Behind (NCLB). NCLB required schools to collect more data to ensure that all students were being served equally (H. R. 1, 2001).
While laudable in principle, the reality was far more complex. A 2009 study conducted by Fordham Law School’s Center on Law and Information Policy found that schools often collected more data than necessary to comply with NCLB and evaluate a school’s overall progress (Reidenberg & Debelak, 2009). In fact, the study noted that state databases included students’ detailed academic, disciplinary, health, and family information, ‘often in a non-anonymous student record’ (Reidenberg & Debelak, 2009). For example, California kept records of students’ parental educational levels, and Iowa and Illinois included data points for students’ criminal histories.
With this mismatch between aspiration and behaviour in the background, parents feared that schools adopting inBloom would end up creating a digital permanent record that tracks, ranks, and categorises students for the rest of their lives (Study session regarding InBloom Inc, 2013). While such a threat was, in itself, ominous, the anxiety was further exacerbated by concern about due process. Parents lacked a clear process for correcting, amending, and erasing incorrect and misleading information contained in the record.
InBloom’s own messaging did little to dissuade fears. Its website listed pages and pages of possible data fields that districts could choose to use (Bulger et al., 2017). While inBloom employees probably perceived this to be a neutral configuration – after all, they were simply listing the fields available without telling districts what specific data should be collected – privacy advocates worried that districts would use all the available fields and collect excessive amounts of data.
The Electronic Privacy Information Center (EPIC) raised concerns that inBloom advertised that it allowed for the creation of ‘principal watch lists’ with no description of why a student might be added to the list. EPIC also criticised inBloom for allowing children to be labelled if they violated ‘norms of behaviour’ (an undefined classification) and categorised as a ‘perpetrator’ or ‘accomplice’ for disciplinary incidents, terms that would normally be used in criminal, not school matters (Study session regarding InBloom Inc, 2013).
Ultimately, inBloom’s leadership erred in their faith that the platform would be seen as sufficiently neutral and that all of the privacy and ethical responsibilities would be transferred to the administrators and teachers at the schools that adopted it. InBloom’s failure to incorporate core Fair Information Principles (another term for the principles we introduced on page 38, like specifying a clear purpose for any data collection and committing to only collect the minimum amount of data needed for that purpose) likely exacerbated privacy concerns.
Public perception was further damaged by inBloom’s privacy policy stating that it could not ‘guarantee the security of the information stored in inBloom or that the information [would] not be intercepted when it [was] being transmitted’ (Ash, 2013). Furthermore, as was the norm for many technology companies, inBloom had a data privacy and security policy that they could unilaterally modify “from time to time, as approved by [an] independent advisory board” (Study session regarding InBloom Inc, 2013). This meant that whatever protections their policy did offer could not be guaranteed; instead, they could change at any time. While these disclaimers and disclosures are standard practice in corporate contracts, this was likely the first time parents had seen these types of clauses. Parents expected that their children’s information would be reasonably protected and expressed unease when it appeared inBloom was dodging responsibility (Ash, 2013).
Finally, and perhaps most importantly, representatives of inBloom did not communicate with parents and advocates effectively (Solove, 2018). An article in ‘Politico’ noted that many educational technology entrepreneurs and school reformers were ‘bewildered by and anxious about the backlash’ against the use of data in education. They had assumed parents would automatically support mining ‘vast quantities of data for insights into what’s working, and what’s not, for individual students and for the education system as a whole,’ and that the benefits were ‘self-evident’ (Simon, 2014). Indeed, the lack of clear answers given to parents and advocates when they raised questions only added to public mistrust and anxiety. Without clear information from inBloom about the platform’s privacy guardrails, parents and advocacy groups narrowly focused on possible abuses of the system.
InBloom lacked a communication plan for addressing the concerns of parents and privacy advocates. It expected school administrators and educators to do the heavy lifting on informing parents about the advantages of the platform. Such overconfidence kept inBloom from providing any communications resources to their education partners. Indeed, they didn’t even inform schools that proactive communication would be necessary. This lack of foresight left schools unprepared to explain how, exactly, the new technology would be beneficial, such as helping parents understand what was happening in their child’s classroom and making it exponentially easier to transfer records when their child transferred schools. Without plausible and positive depictions of how inBloom could responsibly be used, parents had no reason to trust that adopting it would be beneficial. Instead, they had plenty of reasons to be concerned that it would be abused and result in too much information about students being collected. And so, with the bad impressions making a larger impact than the positive ones, every state and district cancelled their contracts with inBloom within one year of its highly anticipated launch (Madda, 2014).
Like inBloom, initiatives around AI in education are often premised on assumptions that ‘everyone’ supposedly agrees with: everyone agrees that data should be easier to share and analyse; everyone agrees that mining data for insights that could help students succeed is worthwhile; everyone wants students to be able to carry their data with them through grades and across districts; everyone wants to take advantage of new technologies to serve students. But if schools or school systems cannot clearly explain how data will be collected, used, shared, and protected, and cannot explain the benefits of initiatives that are based on new data collection or sharing, it will not matter how much that initiative could help students, how much the initiative solves a problem, or whether the initiative has privacy and security built-in. When there is not enough information about an endeavour and privacy and ethics are on the line, people are likely to assume the worst.
Case Study 2 – Mount St. Mary’s
As with the previous case study, this one does not involve the use of AI. Nevertheless, it’s a remarkable cautionary tale for educators considering adopting AI. This is because the fiasco that occurred at Mount St. Mary’s University revolves around predictive analytics, a use of big data that routinely applies AI. Moreover, the problem with how predictive analytics were used at Mount St. Mary’s gets to the heart of a fundamental issue in education itself – equity. The case of Mount St. Mary’s University shines a spotlight on how privacy and ethical guidelines are not only necessary for managing optics, but also for ensuring that the underlying goal of helping each and every student lies at the foundation of all educational initiatives.
Most educators would like to believe that the fundamental goal of education – and associated jobs – is to help every student succeed. It is the guiding ethos of the teaching profession and the true purpose of the work that many view as a vocational calling. However, in the real world, idealism and pragmatism regularly clash. Teaching a diverse student body inevitably requires making compromises and accepting trade-offs. For example, the wellbeing of the majority of students – the students who use the fewest resources and need the fewest interventions – may be prioritised over students with disabilities and students of lower socioeconomic status, who may need more resources and attention. Further complications arise when schools risk losing funding due to lacklustre test scores, low graduation rates and high levels of disciplinary incidents.
While a strictly utilitarian view might justify schools prioritising the interests of the majority instead of trying to apply limited resources equally to everyone, most educators would be repulsed by the prospect of giving up entirely on some children in order to serve the good of the many. If society transitions towards an increasingly data-driven educational model that focuses on maximising efficiency and places increasing priority on AI services, the tension between what is aspirational and what is possible will become more evident.
Mount St. Mary’s University is the second-oldest Catholic university in the United States. In 2014, a private equity CEO and entrepreneur (Svrluga, 2016) was hired as Mount St. Mary’s new president. His goal was to “raise a lot of capital and … start the university on a more aggressive growth trajectory” (Bowie, 2014). With the school board’s approval, he planned to increase the university’s first-to-second year retention rates. Increasing retention rates is significant because the ‘U.S. News and World Report’, an influential publication that ranks colleges and universities, weighs ‘average freshman retention rate’ as one of its highest evaluative categories (Morse, 2013). Schools ranked highly are perceived as prestigious and, as a result, attract quality applicants. Furthermore, alumni can leverage the university’s high profile and ranking when looking for jobs.
During the fall of 2015, Mount St. Mary’s freshmen were asked to take a survey that the president’s office stated would help the school ‘develop better advanced metrics for accepting students’ (Mount President’s attempt, 2016). The survey’s introduction described it as a ‘very valuable tool that will help [students] discover more about themselves’ (Svrluga, 2019). The survey also purported to be ‘based on some of the leading thinking in the area of personal motivation and key factors that determine motivation, success, and happiness’ (Mount President’s Attempt, 2016). Crucially, students were told that ‘there are no wrong answers’ (Svrulga, 2019).
Some questions were innocuous, asking whether students might be interested in certain majors if the school added them. Others were extremely sensitive, asking whether the student had a learning disability, whether a close friend or family member had died in the past year, or whether the student felt they ‘could not shake off the blues, even with help from … family and friends’ (Svulga, 2019).
Unbeknownst to the students and most faculty members, Mount St. Mary’s president planned to use the survey results to inflate the school’s retention rates by asking select students to leave the university (Schisler & Golden, 2016). The administration would contact some of the students whose survey results labelled them at risk of leaving the university and offer them a tuition refund if they dropped out before the federal reporting deadline for the school’s first-year enrolment. When some faculty protested, the president stated, “[t]his is hard for you because you think of the students as cuddly bunnies, but you can’t. You just have to drown the bunnies … put a Glock to their heads” (Schisler & Golden, 2016).
Despite his confidence, the plan failed. The faculty refused to identify the quota of twenty to twenty five students to talk into dropping out, the student newspaper reported on the scheme, and, eventually, enough outrage resulted that the president resigned (Johnson, 2017).
However, he continued to maintain that he was doing the right thing for both the college and students who were likely to fail. According to an op-ed he wrote in ‘The Washington Post’:
‘[r]ather than continuing to collect exorbitant sums of money from the families of continually failing students, the kindest, most responsible option for institutions may be to return students their paid-in tuition and offer guidance for other paths [such as] other universities or technical schooling . . . many students aren’t always willing to raise their hand and say, “I need help.” So, it is our obligation to identify warning signs that can appear as early as a student’s first semester that the academics and college life is not the right fit’ (Svrluga, 2017).
But a sociological analysis of this case found that the survey questions lacked an ‘established connection to educational settings’ and, further, lacked ‘evidence of any effort by the university to test validity or reliability’ to ‘measure of the likelihood of academic success’ (Johnson, 2017). The questions focused largely on ‘non-cognitive characteristics of students’, which included sections on ‘resilience and grit, personality inventories...religious beliefs’ and even included a section for evaluating students for clinical depression (Johnson, 2017).
Another problem with this calculus – beyond the lack of scientific rigour underlying the survey and prior statements where he had expressed anything but sympathy for struggling students – is that the underlying assumptions were not predicated upon universally shared assumptions. While his choice was to push students who seem to have a higher likelihood of failure out of the university, there were other choices, including providing students with more support to prevent them from failing. One first-generation Mount St. Mary’s student interviewed after the president resigned described her first year as “a struggle for me, it was traumatic … [e]very day I would call my mom, sobbing, ‘I don’t think I can do this.’” Fortunately, with a faculty advisor’s help, she persisted, and was a successful third-year student at the time of the interview. “If somebody would have told me when I was a freshman, ‘this is not a good fit for you, you should probably go,’ I would have gone” (Scott, 2019).
No matter how much information a school has about students, and no matter how smart a human or machine’s analysis of it becomes, it is impossible to predict with 100% certainty who will decide to drop out of college. As society aims to create more educational opportunities for lower income and minority students, we should not lose sight of the fact that, since fewer students like them have historically succeeded, predictive algorithms trained on historical data will label these students less likely to succeed here and now. Imagine how such analytics would have rated the chances of women graduating from medical school when universities first began admitting women.
Beyond the potential for discrimination, automated decision-making processes like predictive analytics are not neutral. They are imbued with the values of their creators. In the case of Mount St. Mary’s, the administration’s values were not aligned with faculty or students. The survey’s wording led students to believe there were no wrong answers, encouraging them to answer truthfully and giving them the impression that their answers would not be used against them.
Consequently, students who took the survey consented to the use of their personal information for the survey’s stated purpose – improving the school and student experience – but not its actual purpose – culling the student body to meet the president’s goals for the university. Not only does this case study illustrate what happens when an administration fails to be transparent with data collection and analysis, but it also illustrates the importance of fairness. Students are the largest stakeholders in the context of student privacy conversations. They deserve complete honesty when it comes to why their data is being collected and how it will be used.
Imagine another universe where Mount St. Mary’s created the exact same survey, but instead of pressuring students to leave, they presented them with opportunities for more guidance counselling, tutoring, or a re-ordered class schedule designed to improve student success. If the administration had adhered to privacy and ethical guardrails – such as maintaining a primary focus on the best interests of each student, data minimisation, equity, and transparency – it is unlikely that this initiative would have become a case study in how predictive analytics could harm students.
Teaching AI and privacy ethics
In the previous sections, we highlighted many interrelated ethical and privacy risks associated with big data and AI. We’ll expand the discussion here and offer suggestions for how instructors can effectively teach some of the topics.
As with all subjects, teachers should design age-appropriate lessons. Until recently, it was nearly impossible to find educational material on AI ethics that weren’t created for university students or professionals. Fortunately, things are starting to change. Blakely Payne, a graduate student at the Personal Robotics Group at the MIT Media Lab, created an important resource that instructors at various levels can use and modify. It’s an open-source, module-based curriculum for middle school students on AI that can be integrated into science and humanities classes. Access the material at MIT Media Lab.
Engaging lessons that combine technical and ethical content
Payne’s curriculum is important for three reasons. First, it covers both technical and ethical concepts. Second, it provides examples that students will find resonant. Third, it encourages students to view AI systems as changeable. By combining technical and ethical education, Payne’s approach provides a model for instructors at all levels to emulate. Including technical information is valuable because it helps students develop the technical literacy needed to understand AI.
For example, students begin by learning the basics – understanding what algorithms are. Payne recognises that a useful entry point is for students to view algorithms as recipes containing instructions for generating outputs after acting upon inputs in designated ways. To make this point in an engaging manner, Payne includes an exercise for kids to brainstorm which instructions an algorithm should follow to make the ‘best’ peanut butter and jelly sandwich. Since the sandwich only has a few ingredients, students might presume it’s a basic task. However, in short order, they’ll realise it’s actually a surprisingly complicated endeavour. Moreover, with help from instructors, students can begin to appreciate that the complexity in this seemingly mundane case actually speaks to deep issues that lie at the heart of many consequential applications of AI.
Making the best peanut butter and jelly sandwich isn’t an endeavour that can be reduced to automating a basic process because what ‘best’ means is undefined. Is it an aesthetic ideal, guiding the algorithm to make the best tasting or the best-looking sandwich? Or should ‘best’ be understood as an ideal about safety, guiding the algorithm to make a sandwich that tastes like peanut butter and jelly, but that kids with peanut allergies can eat without getting sick? By helping students appreciate that the definition of concepts like ‘best’ are rooted in ethical values with diverse interpretations, they can be guided towards the eureka moment of grasping that programming algorithms inherently comes with the risk of, to continue with the culinary metaphor, baking in questionable preferences and unfair biases. Becoming aware of this risk – which fundamentally links technical and ethical components of AI – is a teachable skill that enhances the broader skillset of ethical attunement, which educators at every grade level should aspire to develop in their students.
Instructors can cover more advanced material by modifying this engaging approach combining technical and ethical lessons. For example, consider the ethical issue of fairness in the context of AI. As we have already noted, the ideal of fairness is mentioned in the OECD privacy guidelines. However, the concept of fairness, like the concept of best, has additional definitions that correspond to a range of ideals. Fairness encompasses everything from equality of opportunity in situations where candidates are applying for jobs and AI plays a role in determining who is and isn’t hired, to equality in outcome in cases where AI helps determine who gets pulled over for questioning during airport security checks (Binns, 2018).
In an ambitious study of prominent AI ethics guidelines developed around the world (including a discussion paper from the Australian Government Department of Industry, Science, Energy and Resources) Anna Jobin, Marcello Lenca, and Effy Vayena discovered a ‘global convergence’ around five ethical principles: ‘transparency, justice, and fairness, non-maleficence, responsibility and privacy’ (Jobin et al., 2019). (Note: The fact that privacy is listed as one of the most fundamental AI ethics issues corroborates the approach we have been taking here of treating ethics and privacy as deeply connected.) The authors succinctly summarise key findings of an extensive literature review that traverses public and private sectors, carefully explain what the most important AI ethics concepts are and why they matter, and identify some of the most important commonalities and differences in how ethical principles are interpreted.
Let’s bring the discussion of fairness back to teachable examples. The controversy surrounding the use of the U.S. Correctional Offender Management Profiling for Alternative Sanctions (COMPAS) system is an example illustrating the difficulty of translating diverse ethical interpretations of fairness into mathematical models. COMPAS, developed by the private company Northpointe, is a recidivism risk assessment system that predicts the likelihood a defendant will re-offend in the future. U.S. judges use COMPAS as an aid in conjunction with other information when deciding whether or not a defendant should be confined to jail or released on bail until the criminal trial takes place. The hope was that the system and others like it could improve justice by helping generate fairer predictions.
COMPAS generates its results from a questionnaire on the defendant’s criminal history and attitudes about crime, explicitly not including race as a variable for analysis. However, investigative reporting by ProPublica found that COMPAS was twice as likely to erroneously flag black defendants as being high-risk for committing future crimes, with the opposite holding for white defendants, who were twice as likely to be flagged as low-risk (Angwin et al., 2016). The higher risk scores generated by COMPAS do, in fact, correlate with the likelihood that someone will be rearrested, and is nearly identical across racial lines – a result that, taken on its own and without regard for broader context, appears fair because predictions about future criminal offences should apply equally to everyone in a risk category regardless of their race. So, why is it that COMPAS is harsher on black defendants? It appears to be because in the U.S. black defendants have historically been rearrested at higher rates than white ones, and proxies for race, such as prior arrests, can lead COMPAS to classify black defendants as higher risks than white defendants (Corbett-Davies et al., 2016). Although COMPAS was designed to analyse risk without racial bias, historical prejudice nevertheless contributed to biased predictions.
It can be more difficult for students to grasp the idea that different, sometimes conflicting, conceptions of fairness exist than appreciating that there are different ways to make the best peanut butter and jelly sandwich. The danger of algorithms using data tainted by histories of injustice, such as de-contextualised statistics that make minorities seem like criminals or poor job candidates, makes issues surrounding AI and fairness especially timely. Modelling educational exercises that follow Payne’s engaging model of combining technical and ethical lessons will help educators effectively communicate both concepts to students. For example, Karen Hao and Jonathan Stray created ‘Can you make AI fairer than a judge?’ for the ‘MIT Technology Review’. It is an interactive online article that explains the main issues in the COMPAS example in an engaging way, encouraging readers to play a courtroom algorithmic game where they explore issues like trying to make the fairest possible risk-prediction algorithm (Hao & Stray, 2019).
Lessons that focus on examples students can relate to
Payne is right to realise that K-12 educators should primarily focus on examples that will resonate with students based on their personal experiences and professional aspirations. Most conversations about AI focus on potential existential risks set in the distant future with technology that does not yet and may never exist.
Payne focuses on relatable examples because she recognises that children are growing up in a digital world increasingly mediated by AI. Algorithms suggest what they should watch, read and listen to. Since kids are a vulnerable demographic, and the technologies they use in and outside of the classroom will shape everything from what they know to who they are and aspire to be, it’s absolutely critical they are empowered to use them wisely and be critical consumers. To this end, Payne includes a module on redesigning YouTube, a platform recently criticised for nudging kids towards dangerous, conspiratorial content (Roose, 2019).
Her lesson plan has students identify the various stakeholders that YouTube impacts and construct an ethical matrix that highlights where their values overlap and conflict. This exercise is ideal for learning how prioritising different stakeholders with different preferences and values can lead to designing recommendation algorithms that perform in different ways. Crucially, this analytic and pedagogical approach can be usefully applied and adapted to cover a range of AI systems that students should be expected to care about.
We expect students will be interested in how AI is used in schools since these applications can directly impact their lives and futures. This makes examples like the Mount St. Mary’s case especially relevant. The scenario can be adapted to K-12 schools considering using AI to predict who will and won’t benefit from participating in a special program.
Students could write an essay that imagines the school administrators proposing the program to an AI ethics board. Students could pretend to be ethics board members, and writing from this perspective, they could determine who the relevant stakeholders are and what values they embody. Specifically, they should decide to approve or reject the proposal based upon considerations related to them, as well as the OECD principles and AI ethics principles that Jobin, Lenca, and Vayena cover.
An important issue for students to grapple with in this context is what definition of fairness is implicated. We already alluded to it in our previous discussion – it’s the problem of statistical discrimination that fails to treat people as individual decision-makers who might or might not behave like other members of the groups they are identified as belonging to (Binns, 2018).
Similarly, we also expect students will be interested in topics that concern how AI will be used to impact the lives of their parents and other family members. Thus, teachers might want to design assignments that have students think carefully about examples like the Australian controversy surrounding Robodebt that we discussed earlier. Students could reflect on how they would feel if their parents were incorrectly flagged by such a system, and how they would design a better system to prevent the injustice from occurring.
Training tomorrow’s responsible leaders today
Since today’s children are tomorrow’s leaders, it’s important to expose them to the profound ethical and privacy consequences of AI as early as possible. This way, as they get older and start working on projects involving AI, they’ll hopefully be inclined to care about ethical issues from the very start of a project. This ethically attuned outlook is preferable to seeing ethics as an afterthought. Making ethically important changes after a project is completed can be burdened by undue expenses or difficulties that could have been avoided through better planning. The ethically attuned outlook is also preferable to passing responsibility for addressing ethical concerns to other bodies, like regulators. Forgoing this responsibility implicitly rests on the outdated and fallacious presumption that scientists and engineers are not at all responsible for how their devices, systems and ideas are applied (Green, 2018).
We believe it is especially important, as Payne declares that “the ultimate goal is to enable students to see artificial intelligence as manipulatable – from a technical and societal standpoint – and to empower students with tools to design AI with ethics in mind.” Pushing this point further, we would like to suggest that students be given the opportunity to consider cases where the most ethical outcome might be to prevent AI from being used in the first place.
Consider the example we previously discussed of students objecting to being required to learn by using a face scanning engagement system. As mentioned, the CEO of a company that makes one of these systems dismissed their concerns as irrelevant because they are out of step with a presumably inevitable technological evolution. In the classroom, students could be given an opportunity to articulate precise objections to an AI-informed product, like the engagement scanner, and brainstorm ways that the product could be changed or policies could be put in place to promote quality education while mitigating against student concerns. Students should also be given the chance to explain if and why changes or policies won’t suffice and how educational goals could be better met without a particular form of AI or possibly without using AI at all.
By helping students consider the full range of possibilities, educators can truly prepare students to meet the AI imperative – an imperative that does not require solving every possible problem with AI.
Acknowledgements
The authors would like to thank the following individuals for their help and support with this article: Sara Collins, Brenda Leong, Jasmine Park, Anisha Reddy, Alexis Shore, and Katherine Sledge.
References
Angwin, J., Larson, J., Kirchner, L., & Mattu, S. (2016, May 23). Machine bias. ProPublica. https://www.propublica. org/article/machine-bias-risk-assessments-in-criminal- sentencing.
Arizona State University. (2018). Connecting the dots at ASU. https://fullcircle.asu.edu/external/connecting-the- dots-at-asu/
Ash, K. (2013, April 16). InBloom aims to increase data flow despite controversy. Education Week. http://blogs. edweek.org/edweek/DigitalEducation/2013/04/inbloom_ aims_to_increase_data_.html
Binns, R. (2018). Fairness in machine learning: Lessons from political philosophy. Proceedings of machine learning research 81, 1-11. http://proceedings.mlr.press/ v81/binns18a/binns18a.pdf
Bowie, L. (2019, May 28) Simon Newman named Mount St. Mary's president. Baltimore Sun. https://www. baltimoresun.com/education/bs-md-mount-st-marys- president-20141208-story.html.
Bulger, M., McCormick, P., & Pitcan, M. (2017). The Legacy of InBloom. Data & Society. https://datasociety.net/pubs/ ecl/InBloom_feb_2017.pdf
Clark, P., Etzioni, O., Khashabi, D., Khot, T., Mishra, B. D., Richardson, K., Sabharwal, A., Schoenick, C., Tafjord, O., Tandon, N., Bhakthavatsalam, S., Groeneveld, D., Guerquin, M., & Schmitz, M. (2019). From ‘F’ to ‘A’ on the N.Y. Regents Science exams: an overview of the Aristo project. Allen Institute for Artificial Intelligence. https:// arxiv.org/pdf/1909.01958.pdf
Corbett-Davies, S., Pierson, E., Feller, A., & Goel, S. (2016, October 17). A computer program used for bail and sentencing decisions was labeled biased against Blacks. It's actually not that clear. The Washington Post. https:// www.washingtonpost.com/news/monkey-cage/ wp/2016/10/17/can-an-algorithm-be-racist-our-analysis-is- more-cautious-than-propublicas/
Dean, L. (2019, November 19). Government announces major robodebt changes. Yahoo! Finance. https:// au.finance.yahoo.com/news/robodebt-shake-up-as- government-backs-down-042716284.html.
Denver Post. (2016, April 29). InBloom enables great teaching tools. The Denver Post. https://www.denverpost. com/2013/07/18/inbloom-enables-great-teaching-tools/
Eveleth, R. (2018, October 29). Facing tomorrow's high- tech school surveillance. Vice. https://www.vice.com/ amp/en_us/article/j53ba3/facial-recognition-school- surveillance-v25n3.
Emerging technology from the arXiv. (2013, October 3) The big data conundrum: how to define it? MIT Technology Review. https://www.technologyreview.com/s/519851/the-big-data-conundrum-how-to-define- it/
Family Online Safety Institute. (2019). Online safety in the age of artificial intelligence. https://www.fosi.org/policy- research/online-safety-artificial-intelligence/
Future of Privacy Forum. (n.d.). Ethics, governance, and compliance resources. https://sites.google.com/fpf.org/ futureofprivacyforumresources/ethics-governance-and- compliance-resources?authuser=1
Gellman, R. (2019). Fair Information Practices: A Basic History. https://bobgellman.com/rg-docs/rg-FIPshistory. pdf
Green, Ben. (2018). Data science as political action: grounding data science in a politics of justice. arXiv preprint. https://arxiv.org/abs/1811.03435
Greenleaf, G., Clarke, R., & Waters, N. (2013). International Data Privacy Standards: A Global Approach (Australian Privacy Foundation Policy Statement). UNSW Law Research Paper 2013(62). https://papers.ssrn.com/sol3/ papers.cfm?abstract_id=2327325
Hao, K., & Stray, J. (2019, October 17). Can you make AI fairer than a judge? Play our courtroom algorithm game. MIT Technology Review. https://www.technologyreview. com/2019/10/17/75285/ai-fairer-than-judge-criminal-risk- assessment-algorithm/
Haw, M. (2019, September 6). Will AI replace university lecturers? Not if we make it clear why humans matter. The Guardian. https://www.theguardian.com/ education/2019/sep/06/will-ai-replace-university-lecturers-not-if-we-make-it-clear-why-humans-matter.
Jobin, A., Marcello I., & Vayena, E. (2019). The global landscape of AI ethics guidelines. Nature Machine Intelligence, 1, 389–99. https://doi.org/10.1038/s42256-019-0088-2
Johnson, J. (2017, February 24). Structural justice in student analytics, or, the silence of the bunnies [Paper presentation]. Digital sociology mini-conference: Eastern Sociological Society annual meeting, Philadelphia. https://the-other-jeff.com/wp-content/ uploads/2017/02/Structural-Justice-in-Learning- Analytics-ESS.pdf
Karp, P. (2019, November 19). Robodebt: government abandons key part of debt recovery scheme in major overhaul. The Guardian. https://www.theguardian.com/ australia-news/2019/nov/19/robodebt-government- abandons-key-part-of-debt-recovery-scheme-in-major- overhaul
Madda, M. (2014, April 21). What InBloom's Shutdown Means for the Industry. EdSurge. https://www.edsurge. com/news/2014-04-21-what-inbloom-s-shutdown- means-for-the-industry
Marcus, G. (2019, November 30). An Epidemic of AI Misinformation. The Gradient. https://thegradient.pub/ an-epidemic-of-ai-misinformation/
Marsh, S. (2019, November 19). Federal Government immediately halts part of Centrelink robodebt scheme. 9News. https://www.9news.com.au/national/centrelink- robodebt-to-be-halted-immediately-debts-frozen/ c9e2dc06-efda-4e58-a9d2-cdd15a43c856
McCarthy, J. (2007). What is artificial intelligence? Stanford University. http://www-formal.stanford.edu/jmc/ whatisai/
Morse, R. (2013, September 9). Best colleges ranking criteria and weights. US News & World Report. https:// web.archive.org/web/20140802233928/http://www. usnews.com/education/best-colleges/articles/2013/09/09/ best-colleges-ranking-criteria-and-weights
Mount President's attempt to improve retention rate included seeking dismissal of 20-25 first-year students. (2016, January 19. FIRE. https://www.thefire.org/mount- presidents-attempt-to-improve-retention-rate-included- seeking-dismissal-of-20-25-first-year-students/
No Child Left Behind Act 2001, H. R. 1, 107th Cong,., 1st Sess (2001).
Sainato, M. (2015, August 19). Stephen Hawking, Elon Musk, and Bill Gates warn about artificial intelligence. Observer. https://observer.com/2015/08/stephen- hawking-elon-musk-and-bill-gates-warn-about-artificial- intelligence/
Schisler, R, & Golden, R. (2016, January 19). Mount President’s attempt to improve retention rate included seeking dismissal of 20-25 first-year students. The Mountain Echo. http://msmecho.com/2016/01/19/mount- presidents-attempt-to-improve-retention-rate-included- seeking-dismissal-of-20-25-first-year-students/
Scott, A. (2019, April 29). Mount St. Mary's president resigns in the wake of ‘bunnies’ comment. Marketplace. https://www.marketplace.org/2016/02/29/ mount-st-marys-u-quest-better-numbers/
Selinger, E. (2019, October 14). Why technologists fail to think of moderation as a virtue and other stories about AI. Los Angeles Review of Books. https://lareviewofbooks. org/article/why-technologists-fail-to-think-of- moderation-as-a-virtue-and-other-stories-about-ai/
Simon, S. (2014, June 8). Big brother: meet the parents. Politico. http://www.politico.com/story/2014/06/internet- data-mining-children-107461.html
Solove, D. (2018, November 30). Why did inBloom die? A hard lesson about education privacy.
TeachPrivacy, https://teachprivacy.com/inbloom-die- hard-lesson-education-privacy/
Svlurga, S. (2016, January 19). University president allegedly says struggling freshmen are bunnies that should be drowned. The Washington Post. https://www. washingtonpost.com/news/grade-point/wp/2016/01/19/ university-president-allegedly-says-struggling- freshmen-are-bunnies-that-should-be-drowned-that-a- glock-should-be-put-to-their-heads
Svrluga, S. (2019, April 29). The freshman survey that rang alarm bells for some at Mount St. Mary's. The Washington Post. https://www.washingtonpost.com/ news/grade-point/wp/2016/02/12/the-freshman-survey- that-rang-alarm-bells-for-some-at-mount-st-marys/
Study session regarding InBloom, Inc. Colorado State Board of Education (2013) (testimony of Khaliah Barnes).
Reidenberg, J., & Debelak, J. (2009). Children's Educational Records and Privacy. Fordham Center on Law and Information Policy. https://www.fordham. edu/info/23830/research/5922/childrens_educational_ records_and_privacy
Roose, K. (2019, February 19). YouTube unleashed a conspiracy theory boom. Can it be contained? The New York Times. https://www.nytimes.com/2019/02/19/ technology/youtube-conspiracy-stars.html